Demand to identify timber evading economic sanctions.
Around 2021, the term “wood shock” began to circulate, succinctly indicating a timber shortage. Triggered by the COVID-19 pandemic in 2020, and further exacerbated by Russia’s invasion of Ukraine from 2022, the market has experienced a timber deficit. While industries reliant on timber have faced significant economic impacts, leading to a constant search for solutions, the situation surrounding Russia and Ukraine has led to the imposition of import bans and increased tariffs on timber from Russia and Belarus as a form of economic sanction. Before the invasion, Russia and Belarus were key timber exporters, making their exclusion critical, though Western countries have prioritized these sanctions from a political standpoint.
Can you identify the country of origin of timber just by looking at it? While professionals might guess correctly if the countries are significantly different, it becomes challenging when timber from regions with similar latitudes or climates is considered. Indeed, there have been instances of timber from Russia and Belarus being smuggled post-sanctions. Thus, a method using scientific techniques to determine the origin based on numerical data has been developed.
A framework for tracing timber following the Ukraine invasion
Origin identification through machine learning.
Traditionally, scientific methods like Stable Isotope Ratio Analysis (SIRA) and Trace Element Analysis (TEA) have been used for origin identification. SIRA measures the ratio of naturally occurring stable isotopes, which vary with environmental conditions, and can thus indicate the region of origin if regional isotope ratios are known. TEA, on the other hand, measures the macro and micronutrients absorbed by plants, which differ based on the environmental conditions of the growth area, allowing for origin determination.
Now, machine learning models using these values for origin determination are being developed, with this research being one of the first large-scale implementations in Europe, requiring regional optimization.
The analysis has made it possible to narrow down the harvesting location to a range of 180-230km. For context, the distance between Tokyo Station and Hamamatsu Station is approximately 214km, suggesting that, in Japan, it might determine whether timber is from the Kanto or Chubu region. This model could potentially identify 40-60% of samples falsely labeled regarding their origin, with a low chance of incorrectly denying the correct origin. While there is room for improvement, this method could significantly reduce the need for costly investigations to detect fraudulent origins, marking an initial step towards addressing evasion of economic sanctions.
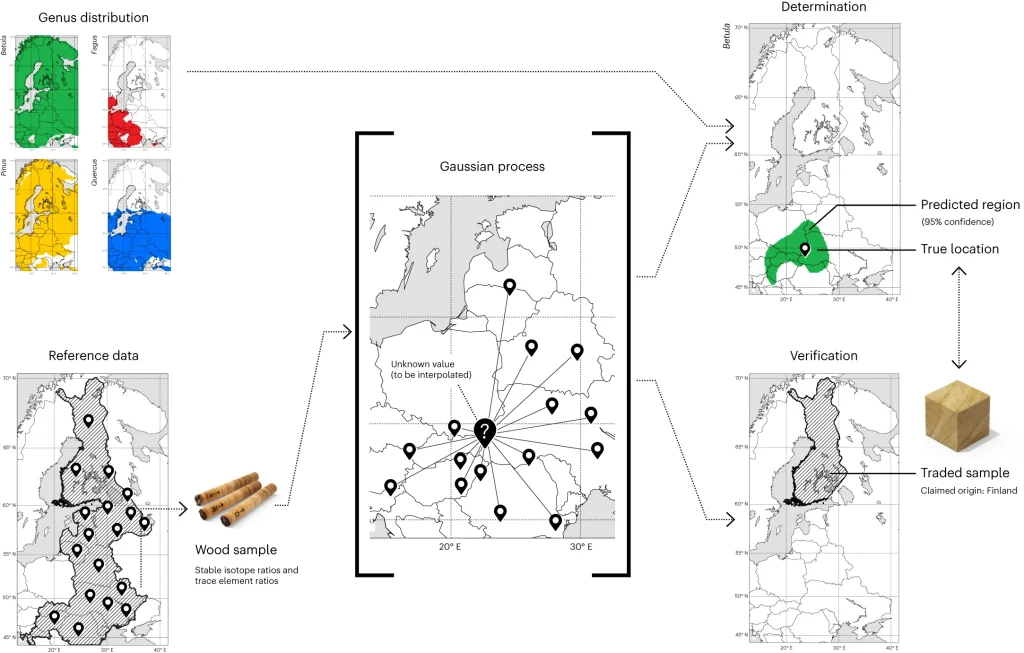
Beyond sanctions, could this have other applications?
The motivation for this study was primarily the economic sanctions resulting from Russia’s invasion of Ukraine. However, the potential applications of this research seem broad.
For instance, identifying regions producing harder or softer timber could help select desired or high-demand timber. Additionally, it could aid in choosing new afforestation sites based on similarities with known timber characteristics.
If the analysis methods and machine learning model construction could be packaged, they might be applicable in other regions as well. As a Japanese, I am curious whether it is possible to identify the origin of Japanese timber.
In the near future, if a “timber database” were created, it might become a crucial source of parameters and data.
コメント